City-scale Car Parking Data Analytics
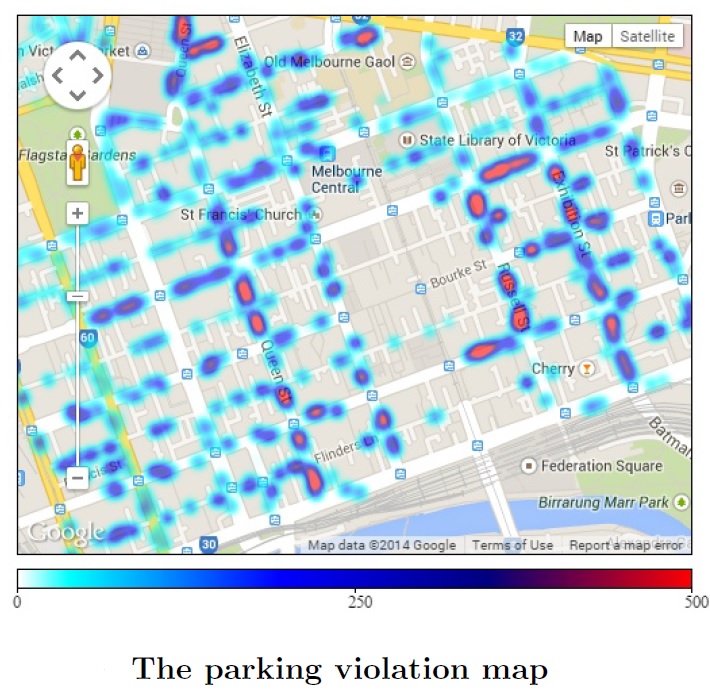
The on-street parking system is an indispensable part of civic projects, as it provides travellers and shoppers with parking spaces. In this project, we use a large real-world dataset with on-street parking sensor data from Melbourne City Council and establish a formulation of the travelling officer problem with a probability-based model. We propose two solutions using a spatio-temporal probability model for parking officers to maximize the number of infringing cars caught with limited time cost. Using real-world parking sensor data and Google Maps road network information, the experimental results show that our proposed algorithms outperform the existing patrolling routes.
This work appears in IEEE Internet of Things Journal.